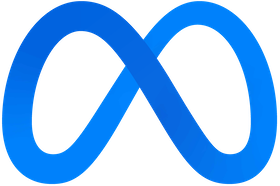
Integration: FAISS
Use a FAISS vector database with Haystack
Faiss is a project by Meta, for efficient vector search. You can use it in your Haystack pipelines with the FAISSDocumentStore
For a detailed explanation on different initialization options of the FAISSDocumentStore
, please visit the
Haystack Documentation and
API Reference. Below are some examples of how you might use it within a Haystack Pipeline.
Installation
pip install farm-haystack[faiss]
or to install FAISSDocumentStore
with GPU support, you may install:
pip install farm-haystack[faiss-gpu]
Usage
Once installed, you can start using FAISS with Haystack by initializing it:
from haystack.document_stores import FAISSDocumentStore
document_store = FAISSDocumentStore()
Writing Documents to FAISSDocumentStore
To write documents to your FAISSDocumentStore
, create an indexing pipeline, or use the write_documents()
function.
For this step, you may make use of the available
FileConverters and
PreProcessors, as well as other
Integrations that might help you fetch data from other resources.
Indexing Pipeline
from haystack import Pipeline
from haystack.document_stores import FAISSDocumentStore
from haystack.nodes import PDFToTextConverter, PreProcessor
document_store = FAISSDocumentStore()
converter = PDFToTextConverter()
preprocessor = PreProcessor()
indexing_pipeline = Pipeline()
indexing_pipeline.add_node(component=converter, name="PDFConverter", inputs=["File"])
indexing_pipeline.add_node(component=preprocessor, name="PreProcessor", inputs=["PDFConverter"])
indexing_pipeline.add_node(component=document_store, name="DocumentStore", inputs=["PreProcessor"])
indexing_pipeline.run(file_paths=["filename.pdf"])
Using Faiss in a Query Pipeline
Once you have documents in your FAISSDocumentStore
, it’s ready to be used in any Haystack pipeline. Such as a Retrieval Augmented Generation (RAG) pipeline. Learn more about
Retrievers to make use of vector search within your LLM pipelines.
from haystack import Pipeline
from haystack.document_stores import FAISSDocumentStore
from haystack.nodes import EmbeddingRetriever, PromptNode
document_store = FAISSDocumentStore()
retriever = EmbeddingRetriever(document_store = document_store,
embedding_model="sentence-transformers/multi-qa-mpnet-base-dot-v1")
prompt_node = PromptNode(model_name_or_path = "gpt-4",
api_key = "YOUR_OPENAI_KEY",
default_prompt_template = "deepset/question-answering-with-references")
query_pipeline = Pipeline()
query_pipeline.add_node(component=retriever, name="Retriever", inputs=["Query"])
query_pipeline.add_node(component=prompt_node, name="PromptNode", inputs=["Retriever"])
query_pipeline.run(query = "What is Haystack?")